Hands-On Neural Networks: A Comprehensive Guide to Deep Learning Architectures, Algorithms, and Hands-On Implementation

Neural networks are a powerful machine learning technique that has achieved remarkable success in a wide range of applications, including image recognition, natural language processing, and speech recognition. In this article, we will provide a comprehensive guide to neural networks, covering the following topics:
- Neural network architectures
- Neural network algorithms
- Hands-on implementation of neural networks
Neural Network Architectures
The architecture of a neural network is the arrangement of its layers and neurons. The most common neural network architecture is the feedforward neural network, which consists of a stack of layers that are connected in a directed manner. Each layer consists of a group of neurons that are connected to the neurons in the previous layer.
4.2 out of 5
Language | : | English |
File size | : | 21292 KB |
Text-to-Speech | : | Enabled |
Screen Reader | : | Supported |
Enhanced typesetting | : | Enabled |
Print length | : | 280 pages |
The input layer of a feedforward neural network receives the input data, and the output layer produces the output of the network. The hidden layers between the input and output layers are responsible for extracting features from the input data and making predictions.
There are many different types of neural network architectures, including convolutional neural networks, recurrent neural networks, and generative adversarial networks. The choice of architecture depends on the specific application for which the neural network is being used.
Neural Network Algorithms
The algorithms that are used to train neural networks are based on gradient descent. Gradient descent is an iterative algorithm that minimizes a loss function by taking steps in the direction of the negative gradient of the loss function.
The loss function is a measure of how well the neural network is performing on the training data. The goal of training is to minimize the loss function so that the neural network makes accurate predictions on new data.
There are many different gradient descent algorithms, including batch gradient descent, stochastic gradient descent, and mini-batch gradient descent. The choice of algorithm depends on the size of the training data set and the computational resources that are available.
Hands-On Implementation of Neural Networks
There are many different ways to implement neural networks. One popular way is to use a deep learning framework, such as TensorFlow or PyTorch. Deep learning frameworks provide a set of tools and libraries that make it easy to build and train neural networks.
In this section, we will provide a hands-on example of how to implement a neural network using TensorFlow. The example will train a neural network to classify images of handwritten digits.
The following code shows how to create a neural network model in TensorFlow:
python model = tf.keras.models.Sequential([ tf.keras.layers.Flatten(input_shape=(28, 28)),tf.keras.layers.Dense(128, activation='relu'),tf.keras.layers.Dropout(0.2),tf.keras.layers.Dense(10, activation='softmax') ])
The model consists of a stack of four layers. The first layer is a Flatten layer, which converts the input image into a one-dimensional array. The next two layers are Dense layers, which are fully connected layers that are used to extract features from the input data. The Dropout layer is used to reduce overfitting. The final layer is a Dense layer with a softmax activation function, which is used to classify the input image.
The following code shows how to train the neural network model:
python model.compile(optimizer='adam', loss='sparse_categorical_crossentropy', metrics=['accuracy'])
model.fit(x_train, y_train, epochs=10)
The compile() method compiles the model by specifying the optimizer, loss function, and metrics that will be used during training. The fit() method trains the model by iterating over the training data and updating the weights of the model's layers.
The following code shows how to evaluate the neural network model:
python model.evaluate(x_test, y_test)
The evaluate() method evaluates the model by computing the loss function and metrics on the test data.
Neural networks are a powerful machine learning technique that can be used to solve a wide range of problems. This article has provided a comprehensive guide to neural networks, covering the following topics:
- Neural network architectures
- Neural network algorithms
- Hands-on implementation of neural networks
We hope that this article has been helpful. If you have any questions, please feel free to leave a comment below.
4.2 out of 5
Language | : | English |
File size | : | 21292 KB |
Text-to-Speech | : | Enabled |
Screen Reader | : | Supported |
Enhanced typesetting | : | Enabled |
Print length | : | 280 pages |
Do you want to contribute by writing guest posts on this blog?
Please contact us and send us a resume of previous articles that you have written.
Book
Novel
Page
Text
Story
Genre
Library
Newspaper
Paragraph
Sentence
Shelf
Glossary
Foreword
Preface
Footnote
Codex
Tome
Bestseller
Memoir
Narrator
Catalog
Card Catalog
Borrowing
Stacks
Study
Research
Scholarly
Lending
Reading Room
Rare Books
Special Collections
Interlibrary
Literacy
Study Group
Thesis
Storytelling
Reading List
Book Club
Theory
Textbooks
John Kubiniec
Paris Anderson
Ron Roecker
Erika Schelby
Naleen Chand Lachan
Herbert Lin
Joanne Bischof
Steven S Smith
Rebecca Scott
Jerry Griffin Ii
Ben Parker
Lope De Vega
Avenged Sevenfold
Amanda Boyarshinov
Shivakumara Palaiahnakote
Sally Rooney
Robert W Valenti
John Muir Laws
Walter R Brooks
Alison Campbell
Light bulbAdvertise smarter! Our strategic ad space ensures maximum exposure. Reserve your spot today!
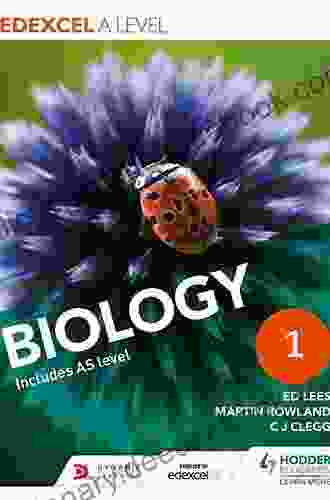

- Caleb LongFollow ·11.6k
- Devon MitchellFollow ·11.1k
- George Bernard ShawFollow ·17.5k
- Jordan BlairFollow ·14k
- Brent FosterFollow ·5.8k
- James HayesFollow ·8.9k
- Isaiah PriceFollow ·5.5k
- Marc FosterFollow ·5.1k
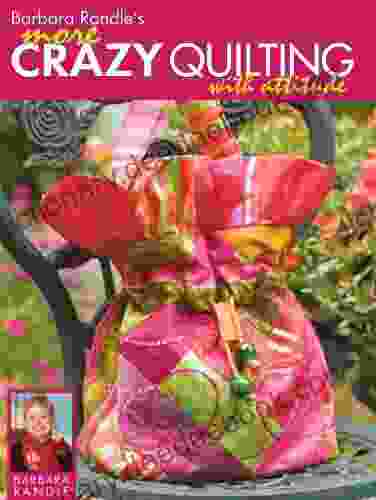

Barbara Randle: More Crazy Quilting With Attitude -...
A Trailblazing Pioneer in...
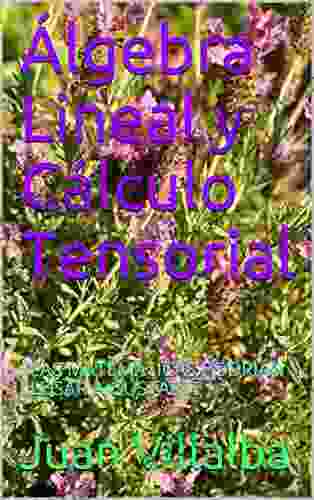

Lapax: A Dystopian Novel by Juan Villalba Explores the...
In the realm of dystopian literature, Juan...
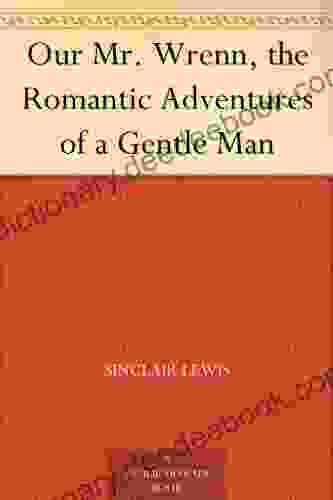

Our Mr. Wrenn: The Romantic Adventures of a Gentle Man
Our Mr. Wrenn is a 1937 novel...
4.2 out of 5
Language | : | English |
File size | : | 21292 KB |
Text-to-Speech | : | Enabled |
Screen Reader | : | Supported |
Enhanced typesetting | : | Enabled |
Print length | : | 280 pages |